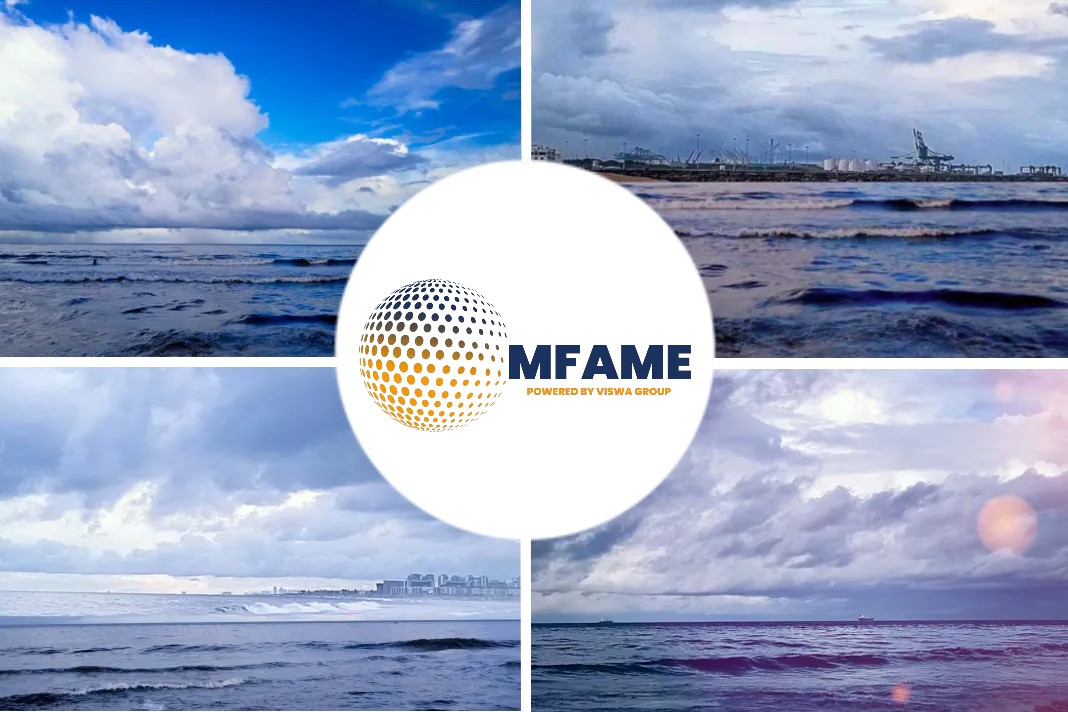
The capacity of Brabant hospitals, including intensive care, will be insufficient in the coming week. Due to overload, approximately 500 to 700 patients will have to be taken to intensive care and other departments in hospitals outside the province, Marcel Visser reported on behalf of the Regional Consultation Acute Care Chain in Brabant in a conversation at the Elisabeth-TweeSteden Hospital in Tilburg. (Photo by Robin Utrecht/SOPA Images/LightRocket via Getty Images)
- Epidemic modeling such as compartment models is the key to develop a pandemic control strategy.
- Compartment models seek to align the infected in groups based on the severity of their disease.
- Factors such as number of diseases in the area and the severity of the disease help in designating specific hot spot areas.
- Much of these strategies need to be revised continuously as new informations and developments pour in.
- The most primary criteria of a good epidemic modeling is flagging off the not so obvious measures like the airport temperature checks.
- An early consensus on epidemic modeling has made it possible to underline a strict containment protocol of COVID19 within 3 months of the disease outbreak.
According to an article published in the Science Magazine, Jacco Wallinga’s computer simulations are about to face a high-stakes reality check as it tries to access the dynamics of pandemic shutdown.
What is it?
The Netherlands has so far chosen a softer set of measures than most Western European countries; it was late to close its schools and restaurants and hasn’t ordered a full lockdown.
In a 16 March speech, Prime Minister Mark Rutte rejected “working endlessly to contain the virus” and “shutting down the country completely.”
Instead, he opted for “controlled spread” of the virus among the groups least at risk of severe illness while making sure the health system isn’t swamped with COVID-19 patients. He called on the public to respect RIVM’s expertise on how to thread that needle.
Wallinga is a mathematician and the chief epidemic modeler at the National Institute for Public Health and the Environment (RIVM), which is advising the Dutch government on what actions, such as closing schools and businesses, will help control the spread of the novel coronavirus in the country.
- Wallinga’s models predict that the number of infected people needing hospitalization, his most important metric, will taper off by the end of the week.
- But if the models are wrong, the demand for intensive care beds could outstrip supply, as it has, tragically, in Italy and Spain.
Huge Responsibility for Researchers
COVID-19 isn’t the first infectious disease scientists have modeled—Ebola and Zika are recent examples—but never has so much depended on their work. Entire cities and countries have been locked down based on hastily done forecasts that often haven’t been peer reviewed. “It has suddenly become very visible how much the response to infectious diseases is based on models,” Wallinga says. For the modelers, “it’s a huge responsibility,” says epidemiologist Caitlin Rivers of the Johns Hopkins University Center for Health Security, who co-authored a report about the future of outbreak modeling in the United States that her center released yesterday.
How good are these public health models?
Just how influential those models are became apparent over the past 2 weeks in the United Kingdom. Based partly on modeling work by a group at Imperial College London, the U.K. government at first implemented fewer measures than many other countries—not unlike the strategy the Netherlands is pursuing. Citywide lockdowns and school closures, as China initially mandated, “would result in a large second epidemic once measures were lifted,” a group of modelers that advises the government concluded in a statement. Less severe controls would still reduce the epidemic’s peak and make any rebound less severe, they predicted.
But on 16 March, the Imperial College group published a dramatically revised model that concluded—based on fresh data from the United Kingdom and Italy—that even a reduced peak would fill twice as many intensive care beds as estimated previously, overwhelming capacity.
- The only choice, they concluded, was to go all out on control measures.
- At best, strict measures might be periodically eased for short periods, the group said (see graphic, below).
- The U.K. government shifted course within days and announced a strict lockdown.
Epidemic modelers are the first to admit their projections can be off. “All models are wrong, but some are useful,” statistician George Box supposedly once said—a phrase that has become a cliché in the field.
Modeling a bleak future
U.K. control measures could be let up once in a while, a model suggests, until demand for intensive care unit (ICU) beds hits a threshold.
What goes behind epidemic modeling?
It’s not that the science behind modeling is controversial.
Wallinga uses a well-established epidemic model that divides the Dutch population into four groups, or compartments in the field’s lingo: healthy, sick, recovered, or dead.
Equations determine how many people move between compartments as weeks and months pass. “The mathematical side is pretty textbook,” he says.
- But model outcomes vary widely depending on the characteristics of a pathogen and the affected population.
- Because the virus that causes COVID-19 is new, modelers need estimates for key model parameters.
- These estimates, particularly in the early days of an outbreak, also come from the work of modelers.
How epidemic modeling helped?
For instance, by late January several groups had published roughly similar estimates of the number of new infections caused by each infected person when no control measures are taken—a parameter epidemiologists call R0.
“This approximate consensus so early in the pandemic gave modelers a chance to warn of this new pathogen’s epidemic and pandemic potential less than 3 weeks after the first Disease Outbreak News report was released by the WHO [World Health Organization] about the outbreak,” says Maia Majumder, a computational epidemiologist at Harvard Medical School whose group produced one of those early estimates.
- Wallinga says his team also spent a lot of time estimating R0 for SARS-Cov-2, the virus that causes COVID-19, and feels sure it’s just over two.
- He is also confident about his estimate that 3 to 6 days elapse between the moment someone is infected and the time they start to infect others.
From a 2017 survey of the Dutch population, the RIVM team also has good estimates of how many contacts people of different ages have at home, school, work, and during leisure. Wallinga says he’s least confident about the susceptibility of each age group to infection and the rate at which people of various ages transmit the virus.
The best estimates come from a study done in Shenzhen, a city in southern China, he says.
How is it done?
- Compartment models assume the population is homogeneously mixed, a reasonable assumption for a small country like the Netherlands.
- Other modeling groups don’t use compartments but simulate the day-to-day interactions of millions of individuals.
- Such models are better able to depict heterogeneous countries, such as the United States, or all of Europe.
- WHO organizes regular calls for COVID-19 modelers to compare strategies and outcomes,
Wallinga says: “That’s a huge help in reducing discrepancies between the models that policymakers find difficult to handle.”
What these models show?
Still, models can produce vastly different pictures. A widely publicized, controversial modeling study published by a group at the University of Oxford argues that the deaths observed in the United Kingdom could be explained by a very different scenario from the currently accepted one.
Rather than SARS-CoV-2 spreading in recent weeks and causing severe disease in a significant percentage of people, as most models suggest, the virus might have been spreading in the United Kingdom since January and could have already infected up to half of the population, causing severe disease only in a tiny fraction. Both scenarios are equally plausible, says Sunetra Gupta, the theoretical epidemiologist who led the Oxford work.
“I do think it is missing from the thinking that there is an equally big possibility that a lot of us are immune,” she says. The model itself cannot answer the question, she says; only widespread testing for antibodies can, and that needs to be done urgently.
Adam Kucharski, a modeler at the London School of Hygiene & Tropical Medicine, says the Oxford group’s new scenario is unlikely. Scientists don’t know exactly how many people develop very mild symptoms or none at all, he says, but data from the Diamond Princess—a cruise ship docked in Yokohama, Japan, for 2 weeks that had a big COVID-19 outbreak—and from repatriation flights and other sources argue against a huge number of asymptomatic cases.
“We don’t know at the moment, is it 50% asymptomatic or is it 20% or 10%,” he says. “I don’t think the question is: Is it 50% asymptomatic or 99.5%.”
Why it didn’t work in the US?
In their review of U.S. outbreak modeling, Rivers and her colleagues note that most of the key players are academics with little role in policy.
They don’t typically “participate in the decision-making processes … they sort of pivot into a new world when an emergency hits,” she says. “It would be more effective if they could be on-site with the government, working side by side with decision makers.”
Rivers argues for the creation of a National Infectious Disease Forecasting Center, akin to the National Weather Service. It would be the primary source of models in a crisis and strengthen outbreak science in “peacetime.”
Policymakers have relied too heavily on COVID-19 models, says Devi Sridhar, a global health expert at the University of Edinburgh. “I’m not really sure whether the theoretical models will play out in real life.” And it’s dangerous for politicians to trust models that claim to show how a little-studied virus can be kept in check, says Harvard University epidemiologist William Hanage. “It’s like, you’ve decided you’ve got to ride a tiger,” he says, “except you don’t know where the tiger is, how big it is, or how many tigers there actually are.”
Flagging the Not So Obvious
Models are at their most useful when they identify something that is not obvious, Kucharski says. One valuable function, he says, was to flag that temperature screening at airports will miss most coronavirus-infected people.
There’s also a lot that models don’t capture. They cannot anticipate, say, the development of a faster, easier test to identify and isolate infected people or an effective antiviral that reduces the need for hospital beds. “That’s the nature of modeling: We put in what we know,” says Ira Longini, a modeler at the University of Florida. Nor do most models factor in the anguish of social distancing, or whether the public obeys orders to stay home.
Recent data from Hong Kong and Singapore suggest extreme social distancing is hard to keep up, says Gabriel Leung, a modeler at the University of Hong Kong.
Both cities are seeing an uptick in cases that he thinks stem at least in part from “response fatigue.”
“We were the poster children because we started early. And we went quite heavy,” Leung says. Now, “It’s 2 months already, and people are really getting very tired.” He thinks both cities may be on the brink of a “major sustained local outbreak”.
Long lockdowns to slow a disease can also have catastrophic economic impacts that may themselves affect public health.
“It’s a three-way tussle,” Leung says, “between protecting health, protecting the economy, and protecting people’s well-being and emotional health.”
The economic fallout isn’t something epidemic models address, Longini says—but that may have to change.
“We should probably hook up with some economic modelers and try to factor that in,” he says.
Did you subscribe to our daily newsletter?
It’s Free! Click here to Subscribe!
Source: Science Magazine